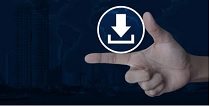
Output = tf.cast(self.prediction > threshold, tf.int32) Loss = tf.reduce_mean(tf.reduce_sum(cross_entropy, axis=1)) Multi label loss: cross_entropy = tf.nn.sigmoid_cross_entropy_with_logits(logits=logits, labels=tf.cast(targets,tf.float32)) I was going through same problem, After some research here is my solution: Y_pred = K.clip(y_pred, K.epsilon(), None) Y_true = K.clip(y_true, K.epsilon(), None) Keras impelmentation def abs_KL_div(y_true, y_pred): $y_=2$ if you have 2 labels for a particular sample. The trick is to model the partition function and the distribution separately, thus exploiting the power of softmax.Ĭonsider your observation vector $y$ to contain $m$ labels. UPDATE (18/04/18): The old answer still proved to be useful on my model. I'm using python and keras for training in case it matters. Looking at the definition of categorical crossentropy I believe it would not apply well to this problem as it will only take into account the output of neurons that should be 1 and ignores the others.īinary cross entropy sounds like it would fit better, but I only see it ever mentioned for binary classification problems with a single output neuron. Now I'm not sure what loss function I should use for this. So my final layer is just sigmoid units that squash their inputs into a probability range 0.1 for every class. I read that for multi-class problems it is generally recommended to use softmax and categorical cross entropy as the loss function instead of mse and I understand more or less why.įor my problem of multi-label it wouldn't make sense to use softmax of course as each class probability should be independent from the other. Each object can belong to multiple classes at the same time (multi-class, multi-label). I'm training a neural network to classify a set of objects into n-classes.
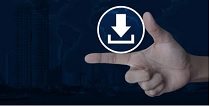